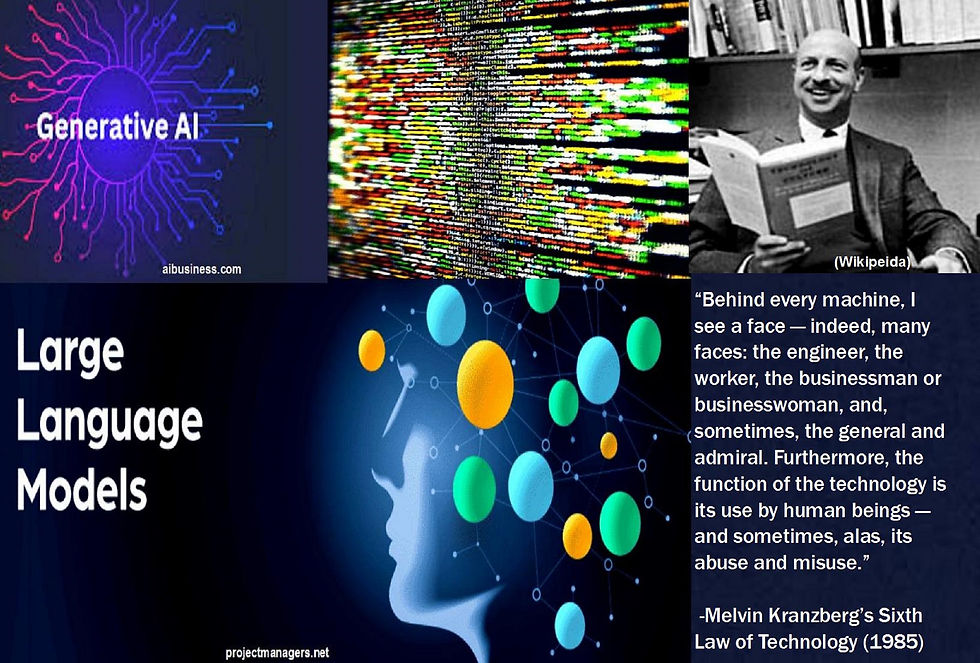
“WAR ON THE ROCKS” By Benjamin Jensen, Yasir Atalan And Ian Reynolds
“No technology is a panacea or poses a risk independent of the people and institutions surrounding its use. Adapting algorithms to support strategic analysis requires studying the people, culture, and bureaucracy as much as it does model performance.”
________________________________________________________________________________
“Prophecy and alarmism about AI are both overblown.
War on the Rocks has been at the center of emerging debates about the roles, missions, and even ethics and morality of the growing integration of AI into the military. Every day, articles emerge highlighting either the game-changing possibilities of generative AI models or the perils associated with them. This trend is particularly acute with respect to large language models that synthesize large volumes of data to generate prompt-based responses that every reader has probably already played with, if not used in a professional setting.
On one side, advocates point to how the ability to synthesize massive information flows will allow militaries to gain a generational relative advantage over adversaries. Knowledge will become the new firepower and algorithms the new decisive points. For example, Alex Karp, the chief executive officer of Palantir, referred to its new Artificial Intelligence Platform as “a weapon that will allow you to win.”
On the other side, some critics highlight the possible “catastrophic risks” of frontier AI models, particularly if increasingly “intelligent” models become unaligned with human goals. Of course, in the context of critical foreign policy and security use cases, the notion of AI models pursuing goals that are distinct from the desires of policymakers calls to mind the possibilities for inadvertent military escalation or unintentional war. For instance, a recent study on a crisis simulation using diplomatic large language model agents showed risks of inadvertent escalation.
However, a purely risk-based reading of these tools tends to miss the social context in which they are developed and deployed and how such contexts can shape model outputs. Moreover, it could lead to conclusions that model outputs are stagnant and fixed rather than fluid and integrated within broader social and organizational structures.
The debate around security integration deserves a more nuanced discussion, as the stakes in security and foreign policy contexts are undeniably high. Yet, the truth about AI lies somewhere between the critics and enthusiasts. The result is that extreme positions tend to miss a key point: Large language models are — to use a famous constructivist phrase — what we make of them. The models are products of the training data that infuses discourses and biases into their application and the people interpreting these outputs. Large language models do not exist in isolation, nor are they tools with concrete, predetermined outcomes. However, the current debate often treats them as such. The social, cultural, and institutional contexts in which these models are developed and deployed should be at the forefront of this discussion. A more useful approach will require studying model outputs and how that will shape any future national security applications with an eye toward the people and bureaucracy surrounding their use.
AI Models and Meaning-Making
Alexander Wendt famously noted that “anarchy is what states make of it.” His basic point was that the “self-help” international system that was commonly assumed to be a timeless feature of relations between states was not simply a natural outcome. It, instead, evolved and stabilized as the result of unfolding social processes that generated broader social structures and identities that shape the conduct of international politics. Neither war nor great power rivalry are constant. Rather, they are constructed and have a different cause and character depending on the context in which leaders opt to use violence in pursuit of politics.
At a basic sociological level, technological developments share aspects of this insight. Technologies do not simply appear from the anti-social void. They are products of human development and, consequently, are embedded within social relations. Such a conceptualization of the relationship between technology and social factors has direct applications for how we assess AI in national security environments. For example, an important research program has already begun to investigate the implications of AI for decision-making, focusing on the importance of contextual factors.
Moreover, others have begun to tease out how AI will interact with military organizations. While not directly associated with a social constructionist approach to international affairs or technology, such studies point to the important social and contextual factors that will shape the relationship between AI and questions of security.
When OpenAI released ChatGPT, it marked the beginning of an era due to its ability to produce meaningful text applicable to various use cases, from email writing to poetry, and script writing to generating programming language. This versatility is rooted in the development processes of Generative AI models. These models are first trained on vast corpuses of data to excel in next-word prediction. Second, a large amount of high-quality human-annotated data is used to instruct the models as part of the fine-tuning process. This later phase is what makes these models generate text that is meaningful and context-dependent. Importantly, while relying on sophisticated algorithms and immense amounts of computing power, these training processes that drive AI outputs are the result of social inputs — both at the level of data scraped from internet sources and in terms of more targeted, human-directed, fine-tuning processes. Ask a model a question about nuclear escalation and it will give you an answer primed for brinkmanship, reflecting both the character of Cold War discussions and the nature of the question.
Despite their capabilities, generative models are not without flaws. Large language models can generate toxic biases and harmful content. This includes, for example, hate speech, abuse, inaccurate information, and stereotypes. The models are just a mirror of the content they are trained on and the question they are asked. They don’t possess inherent values unless they are coded in, and even then, context matters.
Consequently, companies and researchers are finding ways to guardrail models to prevent them from producing undesired or harmful content. One way of doing this is using human-annotated data or prompting to align the models to act in the way that is desired. Additionally, a significant portion of research focuses on finding ways to limit the “biases” of these models. All of these efforts put humans at the center enacting judgment and helping to align model-generated insights with larger questions about politics, morality, and ethics. There is no such thing as non-human strategy, just algorithms eager to answer the questions we ask. This fact puts a premium on knowledge curation and deciding what data counts and how best to weigh different datasets. You don’t want your military decision-making AI trained on Call of Duty chats or romanticized “man on horseback” portrayals of military genius, especially when the subjects are morally repugnant Confederate generals and Nazi leaders.
Guardrails have been somewhat successful to date. This is why we see quite pacifist answers when asked about security-related topics and why users are frequently confronted with limitations when generating images of real people. This caution is necessary, as these models can be harmful. However, some research has shown that models can fail these safety measures. With low-cost adversarial tuning, models can easily generate harmful content. Moreover, these guardrails are based on user prompts and do not consider context. For example, questions about narcotics may be acceptable if the user were a professional in drug enforcement. This is why evaluating a model’s “marginal risks” in use case scenarios is an emerging best practice in model evaluation — or in other words, the difference in risk between whether someone trying to create a bomb leveraged a large language model or simply conducted a standard internet search.
Furthermore, there will be military situations in which commercial guardrails are unwarranted or even dangerous. If an order is lawful and unethical, a strike team doesn’t need to hear why a course of action is inappropriate based on guardrails developed for the general public. Again, context and the human ability to understand and adapt algorithmic reasoning to new situations will prove essential in future war.
Despite the clear issues stemming from model biases, from an epistemological point of view, an “unbiased” or “perfect” model is not possible given that these models are based on training data produced by fallible — and biased — humans who operate within larger sets of norms and social structures. The result is that technological products themselves are infused with certain values that reflect social phenomenon. In practical terms, this means there can only be an “acceptable” level of bias given a model’s use case and context. Moreover, safeguards will need to be adaptable since social norms do not stay fixed and can be contested. Not only that, for each society with their own cultural backgrounds, there will be efforts to guide models to align with certain cultures and prevailing discourses. The future is going to get weird as a mix of politics, culture, and economics skews both guardrail parameters and available training data as seen in the challenges OpenAI experienced due to internet restrictions in China.
In the military context, these factors must be more carefully managed. This involves ensuring the model’s outputs are factually correct and predictable within use case parameters. Yet, a recent study showed that these models can show escalatory behavior inadvertently. This is obviously not a desirable outcome. These concerns should not be overly worrisome as the parameters of training data determine model outputs. For example, when a model shows escalatory behavior in a crisis scenario, it means that the models’ data inputs were more escalatory than expected or desired. Conversely, if you instruct the model to act in a de-escalatory way, it will try to find ways to de-escalate the given scenario. Just because some models show dangerous and escalatory tendencies, it does not mean all models are like this; some can be more de-escalatory based on factors embedded into the model output parameters. Therefore, any model integrated into certain systems should be carefully instructed and tested to have the “right” output parameters for that specific use case.
As a result, we are not able to integrate these models into systems in a monolithic and transformative way due to their subjective nature. These models are not reliable enough to act on their own and they lack strategic reasoning. In fact, it is not universally accepted that the ultimate “end goal” is for these models to function autonomously. Yet, with specified, detailed, and goal-oriented training processes for specific use cases, national security enterprises can make them useful for their organizations.
AI Is Not a Destabilizing Factor … Necessarily
Hype and alarmism are not new; there have always been cautionary tales against emerging technologies alongside promises of revolutionary potential. Particularly, discussions on AI integration into military systems often center on the following question: “Are AI systems inherently dangerous or escalatory?” This question, however, is rather broad and difficult to answer. Rapid conclusions about the inherently escalatory nature of these AI capabilities are misleading. We argue that AI is not necessarily a destabilizing factor. A recent study from the Center for Strategic and International Studies attempted to understand how the intelligence gap on AI capabilities between two nuclear rival countries shapes crisis management and deterrence through a wargame exercise. The goal of the study was to make inferences about players’ risk perceptions by leveraging the variation in information about adversaries’ AI capabilities. Despite valid concerns about AI and machine learning and nuclear escalation, the study found no statistically significant differences between the treatments regarding how players assessed the risk of escalation.
The study showed that when AI entered as a factor in the decision-making process, a lot of countering thought processes were in play throughout the game. At times, national security experts who took part in the wargame considered that adversaries’ AI capabilities could skew the information, leading them to evaluate the team’s behavior as escalation. For example, at one point decision-makers increased intelligence, surveillance, and reconnaissance deployments in adversary regions to offset the information gap. However, in the same phase, fearing misinterpretation by adversaries’ unknown AI capabilities, they took a strong de-escalatory approach in diplomacy to communicate their peaceful intentions. In other words, decision-makers found alternative ways to manage the crisis without escalating, despite the uncertainty about AI’s capabilities. In short, we humans adapt.
The Importance of Social Context
AI and large language model application in foreign policy and security contexts has promise, but they are not magic and must be wisely integrated with an eye toward the social context in which it is developed and deployed. A practical step forward would be substantively educating national security professionals about the properties of models discussed here. Foremost, they are the result of socio-technical processes that will shape model outputs. If humans endeavor to build models to escalate, they likely will. If we build models to show restraint, AI-enabled decision processes could push toward de-escalation. Here, social factors will matter just as much as technological ones. This includes how organizational pressures in security- and foreign policy–related bureaucracies will shape the training parameters of models and — if the time comes — how this technology will be integrated into the everyday practices of organizations. Importantly, the answers to these sorts of questions will determine the boundaries for how AI will shape war and conflict. Moreover, it will be critical to instruct the national security and foreign policy communities on model failure modes and encourage them to find productive ways to combine expert judgment with AI-enabled data processing capabilities.
Second, the tendency to conflate AI and machine learning with autonomy is misguiding the policy debate considering these systems. We are not talking about fully autonomous systems, which increase the risk profile dramatically. Current capabilities still necessitate human centrality in the decision-making process within the military domain. The national security community needs to avoid worrying about Skynet, alongside other technology-related threat inflation, and start building applications that help people navigate the massive volume of information that is already overwhelming staff and decision-makers during a crisis.
Finally, large language models are not geniuses; they mimic us, and their maximum benefits can be achieved only if these models are integrated into systems using a modular approach. A series of wargaming and tabletop exercises with these models showed that they can be useful but only if their use cases are clearly defined in a narrow fashion and if their role in the workflow is clearly delineated. These models cannot strategically reason, but they can navigate vast amounts of information quickly. This capability can be highly useful during military training. In wargames, they can increase efficiency and provide valuable insights with perception testing, recreating historical cases, and summarizing vast amounts of information and data. Again, these models are what you make of them. In other words, they should not be relied upon without significant experimentation, and they must be customized for specific use cases to maximize their contributions. Ethical considerations and the risks of biases and unintended consequences must also be continually addressed. Human oversight remains essential, as AI systems should augment rather than replace decision-making.”
ABOUT THE AUTHORS
Benjamin Jensen, PhD is the Petersen Chair of Emerging Technology at the Marine Corps University and a professor in the School of Advanced Warfighting as well as a senior fellow at the Center for Strategic and International Studies. He is the host of the new War on the Rocks podcast, Not the AI You Are Looking For.
Yasir Atalan and Ian Reynolds, PhD, are researchers in the CSIS Futures Lab where they lead a project building baseline large language models on international relations and strategy using Scale AI’s Donovan platform.
Comments